Delta Lake коннектор#
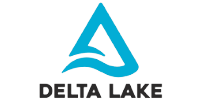
Примечание
Ниже приведена оригинальная документация Trino. Скоро мы ее переведем на русский язык и дополним полезными примерами.
The Delta Lake connector allows querying data stored in the Delta Lake format, including Databricks Delta Lake. The connector can natively read the Delta Lake transaction log and thus detect when external systems change data.
Requirements#
To connect to Databricks Delta Lake, you need:
Tables written by Databricks Runtime 7.3 LTS, 9.1 LTS, 10.4 LTS, 11.3 LTS, 12.2 LTS and 13.3 LTS are supported.
Deployments using AWS, HDFS, Azure Storage, and Google Cloud Storage (GCS) are fully supported.
Network access from the coordinator and workers to the Delta Lake storage.
Access to the Hive metastore service (HMS) of Delta Lake or a separate HMS, or a Glue metastore.
Network access to the HMS from the coordinator and workers. Port 9083 is the default port for the Thrift protocol used by the HMS.
Data files stored in the Parquet file format on a supported file system.
General configuration#
To configure the Delta Lake connector, create a catalog properties file
etc/catalog/example.properties
that references the delta_lake
connector.
You must configure a metastore for metadata.
You must select and configure one of the supported file systems.
connector.name=delta_lake
hive.metastore.uri=thrift://example.net:9083
fs.x.enabled=true
Replace the fs.x.enabled
configuration property with the desired file system.
If you are using AWS Glue as your metastore, you
must instead set hive.metastore
to glue
:
connector.name=delta_lake
hive.metastore=glue
Each metastore type has specific configuration properties along with general metastore configuration properties.
The connector recognizes Delta Lake tables created in the metastore by the Databricks runtime. If non-Delta Lake tables are present in the metastore as well, they are not visible to the connector.
File system access configuration#
The connector supports accessing the following file systems:
You must enable and configure the specific file system access. Legacy support is not recommended and will be removed.
Delta Lake general configuration properties#
The following configuration properties are all using reasonable, tested default values. Typical usage does not require you to configure them.
Property name |
Description |
Default |
---|---|---|
|
Frequency of checks for metadata updates equivalent to transactions to update the metadata cache specified in duration. |
|
|
The maximum number of Delta table metadata entries to cache. |
|
|
Amount of memory allocated for caching information about files. Must be
specified in data size values such as |
|
|
Caching duration for active files that correspond to the Delta Lake tables. |
|
|
The compression codec to be used when writing new data files. Possible values are:
The equivalent catalog session property is |
|
|
Maximum number of partitions per writer. |
|
|
Hide information about tables that are not managed by Delta Lake. Hiding only applies to tables with the metadata managed in a Glue catalog, and does not apply to usage with a Hive metastore service. |
|
|
Enable write support for all supported file systems. Specifically, take note of the warning about concurrency and checkpoints. |
|
|
Default integer count to write transaction log checkpoint entries. If the
value is set to N, then checkpoints are written after every Nth statement
performing table writes. The value can be overridden for a specific table
with the |
|
|
Name of the catalog to which |
|
|
Enable writing row statistics to checkpoint files. |
|
|
Enable pruning of data file entries as well as data file statistics columns
which are irrelevant for the query when reading Delta Lake checkpoint files.
Reading only the relevant active file data from the checkpoint, directly
from the storage, instead of relying on the active files caching, likely
results in decreased memory pressure on the coordinator. The equivalent
catalog session property is |
|
|
Duration to wait for completion of dynamic
filtering during split generation. The equivalent
catalog session property is |
|
|
Enables Table statistics for performance
improvements. The equivalent catalog session property is
|
|
|
Enable statistics collection with ANALYZE and use of extended
statistics. The equivalent catalog session property is
|
|
|
Enable collection of extended statistics for write operations. The
equivalent catalog session property is
|
|
|
Maximum number of metastore data objects per transaction in the Hive metastore cache. |
|
|
Store table comments and colum definitions in the metastore. The write permission is required to update the metastore. |
|
|
Number of threads used for storing table metadata in metastore. |
|
|
Whether schema locations are deleted when Trino can’t determine whether they contain external files. |
|
|
Time zone for Parquet read and write. |
JVM default |
|
Target maximum size of written files; the actual size could be larger. The
equivalent catalog session property is |
|
|
Use randomized, unique table locations. |
|
|
Enable to allow users to call the |
|
|
Minimum retention threshold for the files taken into account for removal by
the VACUUM procedure. The equivalent catalog session
property is |
|
|
Set to |
|
Catalog session properties#
The following table describes catalog session properties supported by the Delta Lake connector:
Property name |
Description |
Default |
---|---|---|
|
The maximum block size used when reading Parquet files. |
|
|
The maximum block size created by the Parquet writer. |
|
|
The maximum page size created by the Parquet writer. |
|
|
The maximum value count of pages created by the Parquet writer. |
|
|
Maximum number of rows processed by the Parquet writer in a batch. |
|
|
Read only projected fields from row columns while performing |
|
Fault-tolerant execution support#
The connector supports Fault-tolerant execution of query processing. Read and write operations are both supported with any retry policy.
Type mapping#
Because Trino and Delta Lake each support types that the other does not, this connector modifies some types when reading or writing data. Data types might not map the same way in both directions between Trino and the data source. Refer to the following sections for type mapping in each direction.
See the Delta Transaction Log specification for more information about supported data types in the Delta Lake table format specification.
Delta Lake to Trino type mapping#
The connector maps Delta Lake types to the corresponding Trino types following this table:
Delta Lake type |
Trino type |
---|---|
|
|
|
|
|
|
|
|
|
|
|
|
|
|
|
|
|
|
|
|
|
|
|
|
|
|
|
|
|
|
|
|
No other types are supported.
Trino to Delta Lake type mapping#
The connector maps Trino types to the corresponding Delta Lake types following this table:
Trino type |
Delta Lake type |
---|---|
|
|
|
|
|
|
|
|
|
|
|
|
|
|
|
|
|
|
|
|
|
|
|
|
|
|
|
|
|
|
|
|
No other types are supported.
Delta Lake table features#
The connector supports the following Delta Lake table features:
Feature |
Description |
---|---|
Append-only tables |
Writers only |
Column invariants |
Writers only |
CHECK constraints |
Writers only |
Change data feed |
Writers only |
Column mapping |
Readers and writers |
Deletion vectors |
Readers and writers |
Iceberg compatibility V1 & V2 |
Readers only |
Invariants |
Writers only |
Timestamp without time zone |
Readers and writers |
Type widening |
Readers only |
Vacuum protocol check |
Readers and writers |
V2 checkpoint |
Readers only |
No other features are supported.
Security#
The Delta Lake connector allows you to choose one of several means of providing authorization at the catalog level. You can select a different type of authorization check in different Delta Lake catalog files.
SQL support#
The connector provides read and write access to data and metadata in Delta Lake. In addition to the globally available and read operation statements, the connector supports the following features:
-
DML, see details for Delta Lake data management
Схемы и таблицы, see details for Delta Lake schema and table management
Time travel queries#
The connector offers the ability to query historical data. This allows to query the table as it was when a previous snapshot of the table was taken, even if the data has since been modified or deleted.
The historical data of the table can be retrieved by specifying the version number corresponding to the version of the table to be retrieved:
SELECT *
FROM example.testdb.customer_orders FOR VERSION AS OF 3
Use the $history
metadata table to determine the snapshot ID of the
table like in the following query:
SELECT version, operation
FROM example.testdb."customer_orders$history"
ORDER BY version DESC
Procedures#
Use the CALL statement to perform data manipulation or
administrative tasks. Procedures are available in the system schema of each
catalog. The following code snippet displays how to call the
example_procedure
in the examplecatalog
catalog:
CALL examplecatalog.system.example_procedure()
Register table#
The connector can register existing Delta Lake tables into the metastore if
delta.register-table-procedure.enabled
is set to true
for the catalog.
The system.register_table
procedure allows the caller to register an
existing Delta Lake table in the metastore, using its existing transaction logs
and data files:
CALL example.system.register_table(schema_name => 'testdb', table_name => 'customer_orders', table_location => 's3://my-bucket/a/path')
To prevent unauthorized users from accessing data, this procedure is disabled by
default. The procedure is enabled only when
delta.register-table-procedure.enabled
is set to true
.
Unregister table#
The connector can remove existing Delta Lake tables from the metastore. Once unregistered, you can no longer query the table from Trino.
The procedure system.unregister_table
allows the caller to unregister an
existing Delta Lake table from the metastores without deleting the data:
CALL example.system.unregister_table(schema_name => 'testdb', table_name => 'customer_orders')
Flush metadata cache#
system.flush_metadata_cache()
Flushes all metadata caches.
system.flush_metadata_cache(schema_name => ..., table_name => ...)
Flushes metadata cache entries of a specific table. Procedure requires passing named parameters.
VACUUM
#
The VACUUM
procedure removes all old files that are not in the transaction
log, as well as files that are not needed to read table snapshots newer than the
current time minus the retention period defined by the retention period
parameter.
Users with INSERT
and DELETE
permissions on a table can run VACUUM
as follows:
CALL example.system.vacuum('exampleschemaname', 'exampletablename', '7d');
All parameters are required and must be presented in the following order:
Schema name
Table name
Retention period
The delta.vacuum.min-retention
configuration property provides a safety
measure to ensure that files are retained as expected. The minimum value for
this property is 0s
. There is a minimum retention session property as well,
vacuum_min_retention
.
Data management#
You can use the connector to INSERT, DELETE, UPDATE, and MERGE data in Delta Lake tables.
Write operations are supported for tables stored on the following systems:
Azure ADLS Gen2, Google Cloud Storage
Writes to the Azure ADLS Gen2 and Google Cloud Storage are enabled by default. Trino detects write collisions on these storage systems when writing from multiple Trino clusters, or from other query engines.
S3 and S3-compatible storage
Writes to Amazon S3 and S3-compatible storage must be enabled with the
delta.enable-non-concurrent-writes
property. Writes to S3 can safely be made from multiple Trino clusters; however, write collisions are not detected when writing concurrently from other Delta Lake engines. You must make sure that no concurrent data modifications are run to avoid data corruption.
Schema and table management#
The Схемы и таблицы functionality includes support for:
ALTER TABLE, see details for Delta Lake ALTER TABLE
The connector supports creating schemas. You can create a schema with or without a specified location.
You can create a schema with the CREATE SCHEMA statement and the
location
schema property. Tables in this schema are located in a
subdirectory under the schema location. Data files for tables in this schema
using the default location are cleaned up if the table is dropped:
CREATE SCHEMA example.example_schema
WITH (location = 's3://my-bucket/a/path');
Optionally, the location can be omitted. Tables in this schema must have a location included when you create them. The data files for these tables are not removed if the table is dropped:
CREATE SCHEMA example.example_schema;
When Delta Lake tables exist in storage but not in the metastore, Trino can be used to register the tables:
CALL example.system.register_table(schema_name => 'testdb', table_name => 'example_table', table_location => 's3://my-bucket/a/path')
The table schema is read from the transaction log instead. If the schema is changed by an external system, Trino automatically uses the new schema.
Предупреждение
Using CREATE TABLE
with an existing table content is disallowed,
use the system.register_table
procedure instead.
If the specified location does not already contain a Delta table, the connector automatically writes the initial transaction log entries and registers the table in the metastore. As a result, any Databricks engine can write to the table:
CREATE TABLE example.default.new_table (id BIGINT, address VARCHAR);
The Delta Lake connector also supports creating tables using the CREATE TABLE AS syntax.
The connector supports the following ALTER TABLE statements.
Replace tables#
The connector supports replacing an existing table as an atomic operation. Atomic table replacement creates a new snapshot with the new table definition as part of the table history.
To replace a table, use CREATE OR REPLACE TABLE
or
CREATE OR REPLACE TABLE AS
.
In this example, a table example_table
is replaced by a completely new
definition and data from the source table:
CREATE OR REPLACE TABLE example_table
WITH (partitioned_by = ARRAY['a'])
AS SELECT * FROM another_table;
ALTER TABLE EXECUTE#
The connector supports the following commands for use with ALTER TABLE EXECUTE.
optimize#
The optimize
command is used for rewriting the content of the specified
table so that it is merged into fewer but larger files. If the table is
partitioned, the data compaction acts separately on each partition selected for
optimization. This operation improves read performance.
All files with a size below the optional file_size_threshold
parameter
(default value for the threshold is 100MB
) are merged:
ALTER TABLE test_table EXECUTE optimize
The following statement merges files in a table that are under 128 megabytes in size:
ALTER TABLE test_table EXECUTE optimize(file_size_threshold => '128MB')
You can use a WHERE
clause with the columns used to partition the table
to filter which partitions are optimized:
ALTER TABLE test_partitioned_table EXECUTE optimize
WHERE partition_key = 1
You can use a more complex WHERE
clause to narrow down the scope of the
optimize
procedure. The following example casts the timestamp values to
dates, and uses a comparison to only optimize partitions with data from the year
2022 or newer:
ALTER TABLE test_table EXECUTE optimize
WHERE CAST(timestamp_tz AS DATE) > DATE '2021-12-31'
ALTER TABLE RENAME TO#
The connector only supports the ALTER TABLE RENAME TO
statement when met with
one of the following conditions:
The table type is external.
The table is backed by a metastore that does not perform object storage operations, for example, AWS Glue.
Table properties#
The following table properties are available for use:
Property name |
Description |
---|---|
|
File system location URI for the table. |
|
Set partition columns. |
|
Set the checkpoint interval in number of table writes. |
|
Enables storing change data feed entries. |
|
Column mapping mode. Possible values are:
Defaults to |
|
Enables deletion vectors. |
The following example uses all available table properties:
CREATE TABLE example.default.example_partitioned_table
WITH (
location = 's3://my-bucket/a/path',
partitioned_by = ARRAY['regionkey'],
checkpoint_interval = 5,
change_data_feed_enabled = false,
column_mapping_mode = 'name',
deletion_vectors_enabled = false
)
AS SELECT name, comment, regionkey FROM tpch.tiny.nation;
Shallow cloned tables#
The connector supports read and write operations on shallow cloned tables. Trino does not support creating shallow clone tables. More information about shallow cloning is available in the Delta Lake documentation.
Shallow cloned tables let you test queries or experiment with changes to a table without duplicating data.
Metadata tables#
The connector exposes several metadata tables for each Delta Lake table. These metadata tables contain information about the internal structure of the Delta Lake table. You can query each metadata table by appending the metadata table name to the table name:
SELECT * FROM "test_table$history"
$history
table#
The $history
table provides a log of the metadata changes performed on
the Delta Lake table.
You can retrieve the changelog of the Delta Lake table test_table
by using the following query:
SELECT * FROM "test_table$history"
version | timestamp | user_id | user_name | operation | operation_parameters | cluster_id | read_version | isolation_level | is_blind_append
---------+---------------------------------------+---------+-----------+--------------+---------------------------------------+---------------------------------+--------------+-------------------+----------------
2 | 2023-01-19 07:40:54.684 Europe/Vienna | trino | trino | WRITE | {queryId=20230119_064054_00008_4vq5t} | trino-406-trino-coordinator | 2 | WriteSerializable | true
1 | 2023-01-19 07:40:41.373 Europe/Vienna | trino | trino | ADD COLUMNS | {queryId=20230119_064041_00007_4vq5t} | trino-406-trino-coordinator | 0 | WriteSerializable | true
0 | 2023-01-19 07:40:10.497 Europe/Vienna | trino | trino | CREATE TABLE | {queryId=20230119_064010_00005_4vq5t} | trino-406-trino-coordinator | 0 | WriteSerializable | true
The output of the query has the following history columns:
Name |
Type |
Description |
---|---|---|
|
|
The version of the table corresponding to the operation |
|
|
The time when the table version became active |
|
|
The identifier for the user which performed the operation |
|
|
The username for the user which performed the operation |
|
|
The name of the operation performed on the table |
|
|
Parameters of the operation |
|
|
The ID of the cluster which ran the operation |
|
|
The version of the table which was read in order to perform the operation |
|
|
The level of isolation used to perform the operation |
|
|
Whether or not the operation appended data |
$partitions
table#
The $partitions
table provides a detailed overview of the partitions of the
Delta Lake table.
You can retrieve the information about the partitions of the Delta Lake table
test_table
by using the following query:
SELECT * FROM "test_table$partitions"
partition | file_count | total_size | data |
-------------------------------+------------+------------+----------------------------------------------+
{_bigint=1, _date=2021-01-12} | 2 | 884 | {_decimal={min=1.0, max=2.0, null_count=0}} |
{_bigint=1, _date=2021-01-13} | 1 | 442 | {_decimal={min=1.0, max=1.0, null_count=0}} |
The output of the query has the following columns:
Name |
Type |
Description |
---|---|---|
|
|
A row that contains the mapping of the partition column names to the partition column values. |
|
|
The number of files mapped in the partition. |
|
|
The size of all the files in the partition. |
|
|
Partition range and null counts. |
$properties
table#
The $properties
table provides access to Delta Lake table configuration,
table features and table properties. The table rows are key/value pairs.
You can retrieve the properties of the Delta
table test_table
by using the following query:
SELECT * FROM "test_table$properties"
key | value |
----------------------------+-----------------+
delta.minReaderVersion | 1 |
delta.minWriterVersion | 4 |
delta.columnMapping.mode | name |
delta.feature.columnMapping | supported |
Metadata columns#
In addition to the defined columns, the Delta Lake connector automatically exposes metadata in a number of hidden columns in each table. You can use these columns in your SQL statements like any other column, e.g., they can be selected directly or used in conditional statements.
$path
Full file system path name of the file for this row.
$file_modified_time
Date and time of the last modification of the file for this row.
$file_size
Size of the file for this row.
Table functions#
The connector provides the following table functions:
table_changes#
Allows reading Change Data Feed (CDF) entries to expose row-level changes
between two versions of a Delta Lake table. When the change_data_feed_enabled
table property is set to true
on a specific Delta Lake table,
the connector records change events for all data changes on the table.
This is how these changes can be read:
SELECT
*
FROM
TABLE(
system.table_changes(
schema_name => 'test_schema',
table_name => 'tableName',
since_version => 0
)
);
schema_name
- type VARCHAR
, required, name of the schema for which the function is called
table_name
- type VARCHAR
, required, name of the table for which the function is called
since_version
- type BIGINT
, optional, version from which changes are shown, exclusive
In addition to returning the columns present in the table, the function returns the following values for each change event:
_change_type
Gives the type of change that occurred. Possible values are
insert
,delete
,update_preimage
andupdate_postimage
.
_commit_version
Shows the table version for which the change occurred.
_commit_timestamp
Represents the timestamp for the commit in which the specified change happened.
This is how it would be normally used:
Create table:
CREATE TABLE test_schema.pages (page_url VARCHAR, domain VARCHAR, views INTEGER)
WITH (change_data_feed_enabled = true);
Insert data:
INSERT INTO test_schema.pages
VALUES
('url1', 'domain1', 1),
('url2', 'domain2', 2),
('url3', 'domain1', 3);
INSERT INTO test_schema.pages
VALUES
('url4', 'domain1', 400),
('url5', 'domain2', 500),
('url6', 'domain3', 2);
Update data:
UPDATE test_schema.pages
SET domain = 'domain4'
WHERE views = 2;
Select changes:
SELECT
*
FROM
TABLE(
system.table_changes(
schema_name => 'test_schema',
table_name => 'pages',
since_version => 1
)
)
ORDER BY _commit_version ASC;
The preceding sequence of SQL statements returns the following result:
page_url | domain | views | _change_type | _commit_version | _commit_timestamp
url4 | domain1 | 400 | insert | 2 | 2023-03-10T21:22:23.000+0000
url5 | domain2 | 500 | insert | 2 | 2023-03-10T21:22:23.000+0000
url6 | domain3 | 2 | insert | 2 | 2023-03-10T21:22:23.000+0000
url2 | domain2 | 2 | update_preimage | 3 | 2023-03-10T22:23:24.000+0000
url2 | domain4 | 2 | update_postimage | 3 | 2023-03-10T22:23:24.000+0000
url6 | domain3 | 2 | update_preimage | 3 | 2023-03-10T22:23:24.000+0000
url6 | domain4 | 2 | update_postimage | 3 | 2023-03-10T22:23:24.000+0000
The output shows what changes happen in which version.
For example in version 3 two rows were modified, first one changed from
('url2', 'domain2', 2)
into ('url2', 'domain4', 2)
and the second from
('url6', 'domain2', 2)
into ('url6', 'domain4', 2)
.
If since_version
is not provided the function produces change events
starting from when the table was created.
SELECT
*
FROM
TABLE(
system.table_changes(
schema_name => 'test_schema',
table_name => 'pages'
)
)
ORDER BY _commit_version ASC;
The preceding SQL statement returns the following result:
page_url | domain | views | _change_type | _commit_version | _commit_timestamp
url1 | domain1 | 1 | insert | 1 | 2023-03-10T20:21:22.000+0000
url2 | domain2 | 2 | insert | 1 | 2023-03-10T20:21:22.000+0000
url3 | domain1 | 3 | insert | 1 | 2023-03-10T20:21:22.000+0000
url4 | domain1 | 400 | insert | 2 | 2023-03-10T21:22:23.000+0000
url5 | domain2 | 500 | insert | 2 | 2023-03-10T21:22:23.000+0000
url6 | domain3 | 2 | insert | 2 | 2023-03-10T21:22:23.000+0000
url2 | domain2 | 2 | update_preimage | 3 | 2023-03-10T22:23:24.000+0000
url2 | domain4 | 2 | update_postimage | 3 | 2023-03-10T22:23:24.000+0000
url6 | domain3 | 2 | update_preimage | 3 | 2023-03-10T22:23:24.000+0000
url6 | domain4 | 2 | update_postimage | 3 | 2023-03-10T22:23:24.000+0000
You can see changes that occurred at version 1 as three inserts. They are
not visible in the previous statement when since_version
value was set to 1.
Производительность#
Данная секция описывает важные улучшения производительности, реализованные в Delta Lake коннекторе.
Поддержка write partitioning.
Локальный дисковый кэш данных#
Коннектор позволяет сохранять часть данных из озера данных на дисках worker-узлов CedrusData для ускорения доступа к ним. Во многих случаях использование локального дискового кэша приводит к кратному ускорению запросов.
При каждом доступе к колонке CedrusData проверяет, были ли закэшированные данные изменены в удаленном источнике. Если обнаружено изменение, локальные данные будут удалены и закэшированы повторно.
CedrusData кэширует метаданные файлов, а также диапазоны записей по мере необходимости. Гранулярность кэширования диапазонов записей зависит от формата:
Для формата Parquet единицей кэширования являются данные колонки внутри row group
Для формата ORC единицей кэширования являются данные колонки внутри stripe
Для включения локального дискового кэша необходимо:
Установить параметр конфигурации каталога
cedrusdata.hive.data-cache.enabled=true
Указать путь к файлу, в котором описаны правила кэширования в JSON формате
Для узлов, которые будут выполнять запросы (все worker-узлы, а также coordinator-узел, запущенный с параметром
node-scheduler.include-coordinator=true
) необходимо также указать путь к директории, в которой CedrusData будет хранить закэшированные данные. Параметр конфигурации:cedrusdata.hive.data-cache.path
Пример конфигурации для координатора, который не выполняет запросы:
cedrusdata.hive.data-cache.enabled=true
cedrusdata.hive.data-cache.rules.file=/path/to/rules.json
Пример конфигурации для worker-узла или координатора, который выполняет запросы (node-scheduler.include-coordinator=true
):
cedrusdata.hive.data-cache.enabled=true
cedrusdata.hive.data-cache.rules.file=/path/to/rules.json
cedrusdata.hive.data-cache.path=file:///path/to/cache/dir
Правила кэширования необходимо задать в отдельном файле в формате:
{
"rules": [
<rule1>,
<rule2>,
...
]
}
Где <rule>
представляет собой отдельное правило в формате:
{
"schema": "<regexp схемы>",
"table": "<regexp таблицы>",
"partition_filter": "<предикат ключа партиционирования таблицы>",
"distribution_mode": "<способ распределения сплитов по узлам>",
"affinity_mode": "<способ привязки сплитов к узлам>",
"affinity_node_count": "<количество узлов на которых может быть обработан сплит>",
"disable_cache": <флаг отключения кэширования>
}
Подробное описание полей правила:
Название |
Описание |
---|---|
|
Обязательное поле.
Задает паттерн для схем в формате Java Pattern.
Например, |
|
Опциональное поле.
Задает паттерн для таблиц в формате Java Pattern.
Например, |
|
Опциональное поле.
Задает фильтр партиции в виде SQL выражения. Выражение может ссылаться на колонки партиции таблицы и использовать стандартные функции.
Выражение не может ссылаться на колонки, которые не входят в ключ партиции, а также содержать подзапросы и вызовы табличных функций.
Вызов функций происходит от имени специального системного пользователя, на которого не распространяются проверки доступа к функциям.
Если значение |
|
Опциональное поле.
Поддерживается только для Hive коннектора.
Задает способ сопоставления сплитов с worker-узлами.
Допустимые значения: |
|
Опциональное поле.
Задает механизм привязки сплита к узлу.
Допустимые значения: |
|
Опциональное поле.
Задает количество узлов на которых может быть выполнен сплит.
Увеличение данного значения приводит к дублированию закэшированных в кластере, но обеспечивает более равномерное распределение нагрузки.
Если |
|
Опциональное поле.
Позволяет отключить кэширование заданных объектов, соответствующих заданным паттернам |
Обработка правил происходит в порядке их указания в файле сверху вниз. Проверка, кэшировать ли данные из текущей таблицы или партиции, завершается, как только найдено первое подходящее правило.
Ниже приведен полный пример файла правил, который включает кэширование для всех таблиц схем s1
и s2
, кроме таблицы s2.excluded_table
,
а также для таблицы s3.partitioned_table
, в которой закэшированы будут только партиции продаж за последний месяц:
{
"rules": [
{
"schema": "s2",
"table": "excluded_table",
"disable_cache": true
},
{
"schema": "s1|s2"
},
{
"schema": "s3",
"table": "partitioned_table",
"partition_filter": "sales_date + interval '1' month <= current_date"
}
]
}
Вы можете изменять содержимое файла без перезапуска узла. Повторное чтение содержимого файла происходит периодически в соответствии с параметром конфигурации
cedrusdata.hive.data-cache.rules.refresh-period
.
Название |
Описание |
Значение по умолчанию |
---|---|---|
|
Использовать ли локальный кэш данных. Параметр сессии: |
|
|
Путь к файлу с правилами кэширования в формате JSON. |
|
|
Как частно повторно считывать правила кэширования из файла, путь к которому задан в |
|
|
Имя часового пояса, в контексте которого происходит вычисление предикатов партиций. |
Имя текущего часового пояса JVM |
|
Размер кэша, в котором хранится решение о кэшировании и скомпилированный предикат партиции (при наличии) для таблиц. Значение 0 отключает кэширование (не рекомендовано). |
1000 |
|
Путь к локальной директории узла, в котором будут сохранены закэшированные данные.
Путь должен быть задан в формате |
|
|
Максимальный размер кэша данных. При превышении размера CedrusData начнет удаление наиболее редко используемых данных. Данный размер учитывает реальный размер данных на диске с учетом возможной компрессии (см. ниже). |
|
|
Максимальное время хранения записи в кэше. По истечении данного времени запись будет удалена из кэша. |
|
|
Режим компрессии закэшированных данных. При отсутствии компрессии данные занимают больше места на диске, но при
этом требуют меньше ресурсов CPU для чтения. При включенной компрессии данные занимают меньше месте на диске, но
каждая операция чтения потребляет больше CPU. Принимайте решение о включении компрессии на основе того, какой
ресурс узла является более дефицитным. Доступные значения: |
|
|
Как часто производить очистку кэша от устаревших записей. Запись считается устаревшей, если истек ее TTL, заданный параметром |
|
|
Сколько записей возвращать движку CedrusData при чтении данных из кэша. Используется для тонкой настройки производительности. В большинстве случаев его изменение не требуется. |
|
|
Максимальное количество операций кэширования удаленных данных. Когда CedrusData обнаруживает, что удаленные данные соответствуют заданным правилам кэширования, но отсутствуют в кэше, происходит асинхронное кэширование данных, которое требует повторное удаленное чтение. Таким образом, при прогреве кэша могут возникать всплески сетевой и дисковой I/O активности, которые могут негативно сказаться на производительности текущих запросов. Для уменьшения негативного эффекта вы можете задать максимальное количество запросов на запись данных в кэш. |
|
|
Размер буфера при чтении данных с диска. Увеличения размера буфера приводит к уменьшению количества IOPS,
требуемых для чтения данных, но увеличивает потребление памяти. Значение по умолчанию должно хорошо
справляться с большинством типичных нагрузок. Настройка данного параметра может быть полезна в облачных
окружениях, которые зачастую ограничивают количество IOPS в секунду. Обратите внимание, что размерность
«килобайт» необходимо указывать как |
|
|
Размер буфера для записи данных на диск. Увеличения размера буфера приводит к уменьшению количества IOPS,
требуемых для записи данных, но увеличивает потребление памяти. Значение по умолчанию должно хорошо
справляться с большинством типичных нагрузок. Настройка данного параметра может быть полезна в облачных
окружениях, которые зачастую ограничивают количество IOPS в секунду. Обратите внимание, что размерность
«килобайт» необходимо указывать как |
|
Для отчистки кэша конкретного каталога воспользуйтесь встроенной процедурой system.cedrusdata.clear_data_cache
.
Единственным аргументом процедуры является название каталога. Следующий запрос очищает кэш каталога my_data_lake
:
CALL system.cedrusdata.clear_data_cache('my_data_lake');
Статистики работы кэша доступны через таблицу JMX коннектор trino.plugin.hive.datacache:name=<имя каталога>,type=hivedatacacheservice
.
Следующий запрос отображает текущие статистики кэша каталога my_data_lake
:
SELECT * FROM jmx."current"."trino.plugin.hive.datacache:name=my_data_lake,type=hivedatacacheservice"
Table statistics#
Use ANALYZE statements in Trino to populate data size and number of distinct values (NDV) extended table statistics in Delta Lake. The minimum value, maximum value, value count, and null value count statistics are computed on the fly out of the transaction log of the Delta Lake table. The cost-based optimizer then uses these statistics to improve query performance.
Extended statistics enable a broader set of optimizations, including join
reordering. The controlling catalog property delta.table-statistics-enabled
is enabled by default. The equivalent catalog session property is statistics_enabled
.
Each ANALYZE
statement updates the table statistics incrementally, so only
the data changed since the last ANALYZE
is counted. The table statistics are
not automatically updated by write operations such as INSERT
, UPDATE
,
and DELETE
. You must manually run ANALYZE
again to update the table
statistics.
To collect statistics for a table, execute the following statement:
ANALYZE table_schema.table_name;
To recalculate from scratch the statistics for the table use additional parameter mode
:
ANALYZE table_schema.table_name WITH(mode = „full_refresh“);
There are two modes available full_refresh
and incremental
.
The procedure use incremental
by default.
To gain the most benefit from cost-based optimizations, run periodic ANALYZE
statements on every large table that is frequently queried.
Fine-tuning#
The files_modified_after
property is useful if you want to run the
ANALYZE
statement on a table that was previously analyzed. You can use it to
limit the amount of data used to generate the table statistics:
ANALYZE example_table WITH(files_modified_after = TIMESTAMP '2021-08-23
16:43:01.321 Z')
As a result, only files newer than the specified time stamp are used in the analysis.
You can also specify a set or subset of columns to analyze using the columns
property:
ANALYZE example_table WITH(columns = ARRAY['nationkey', 'regionkey'])
To run ANALYZE
with columns
more than once, the next ANALYZE
must
run on the same set or a subset of the original columns used.
To broaden the set of columns
, drop the statistics and reanalyze the table.
Disable and drop extended statistics#
You can disable extended statistics with the catalog configuration property
delta.extended-statistics.enabled
set to false
. Alternatively, you can
disable it for a session, with the catalog session property extended_statistics_enabled
set to false
.
If a table is changed with many delete and update operation, calling ANALYZE
does not result in accurate statistics. To correct the statistics, you have to
drop the extended statistics and analyze the table again.
Use the system.drop_extended_stats
procedure in the catalog to drop the
extended statistics for a specified table in a specified schema:
CALL example.system.drop_extended_stats('example_schema', 'example_table')
Memory usage#
The Delta Lake connector is memory intensive and the amount of required memory grows with the size of Delta Lake transaction logs of any accessed tables. It is important to take that into account when provisioning the coordinator.
You must decrease memory usage by keeping the number of active data files in
the table low by regularly running OPTIMIZE
and VACUUM
in Delta Lake.
Memory monitoring#
When using the Delta Lake connector, you must monitor memory usage on the coordinator. Specifically, monitor JVM heap utilization using standard tools as part of routine operation of the cluster.
A good proxy for memory usage is the cache utilization of Delta Lake caches. It
is exposed by the connector with the
plugin.deltalake.transactionlog:name=<catalog-name>,type=transactionlogaccess
JMX bean.
You can access it with any standard monitoring software with JMX support, or use the JMX коннектор with the following query:
SELECT * FROM jmx.current."*.plugin.deltalake.transactionlog:name=<catalog-name>,type=transactionlogaccess"
Following is an example result:
datafilemetadatacachestats.hitrate | 0.97
datafilemetadatacachestats.missrate | 0.03
datafilemetadatacachestats.requestcount | 3232
metadatacachestats.hitrate | 0.98
metadatacachestats.missrate | 0.02
metadatacachestats.requestcount | 6783
node | trino-master
object_name | io.trino.plugin.deltalake.transactionlog:type=TransactionLogAccess,name=delta
In a healthy system, both datafilemetadatacachestats.hitrate
and
metadatacachestats.hitrate
are close to 1.0
.
Table redirection#
Trino offers the possibility to transparently redirect operations on an existing table to the appropriate catalog based on the format of the table and catalog configuration.
In the context of connectors which depend on a metastore service (for example, Hive коннектор, Iceberg коннектор and Delta Lake коннектор), the metastore (Hive metastore service, AWS Glue Data Catalog) can be used to accustom tables with different table formats. Therefore, a metastore database can hold a variety of tables with different table formats.
As a concrete example, let’s use the following simple scenario which makes use of table redirection:
USE example.example_schema;
EXPLAIN SELECT * FROM example_table;
Query Plan
-------------------------------------------------------------------------
Fragment 0 [SOURCE]
...
Output[columnNames = [...]]
│ ...
└─ TableScan[table = another_catalog:example_schema:example_table]
...
The output of the EXPLAIN
statement points out the actual
catalog which is handling the SELECT
query over the table example_table
.
The table redirection functionality works also when using fully qualified names for the tables:
EXPLAIN SELECT * FROM example.example_schema.example_table;
Query Plan
-------------------------------------------------------------------------
Fragment 0 [SOURCE]
...
Output[columnNames = [...]]
│ ...
└─ TableScan[table = another_catalog:example_schema:example_table]
...
Trino offers table redirection support for the following operations:
Table read operations
Table write operations
Table management operations
Trino does not offer view redirection support.
The connector supports redirection from Delta Lake tables to Hive tables
with the delta.hive-catalog-name
catalog configuration property.
Performance tuning configuration properties#
The following table describes performance tuning catalog properties specific to the Delta Lake connector.
Предупреждение
Performance tuning configuration properties are considered expert-level features. Altering these properties from their default values is likely to cause instability and performance degradation. It is strongly suggested that you use them only to address non-trivial performance issues, and that you keep a backup of the original values if you change them.
Property name |
Description |
Default |
---|---|---|
|
Minimum size of query predicates above which Trino compacts the predicates. Pushing a large list of predicates down to the data source can compromise performance. For optimization in that situation, Trino can compact the large predicates. If necessary, adjust the threshold to ensure a balance between performance and predicate pushdown. |
|
|
The target number of buffered splits for each table scan in a query, before the scheduler tries to pause. |
|
|
Sets the maximum number of splits used per second to access underlying storage. Reduce this number if your limit is routinely exceeded, based on your filesystem limits. This is set to the absolute maximum value, which results in Trino maximizing the parallelization of data access by default. Attempting to set it higher results in Trino not being able to start. |
|
|
Sets the largest data size for a single read section
assigned to a worker after |
|
|
A decimal value in the range (0, 1] used as a minimum for weights assigned to each split. A low value might improve performance on tables with small files. A higher value might improve performance for queries with highly skewed aggregations or joins. |
|
|
Read only projected fields from row columns while performing |
|
|
Set to |
|
File system cache#
The connector supports configuring and using file system caching.