Iceberg коннектор#
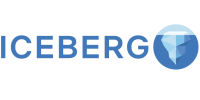
Примечание
Ниже приведена оригинальная документация Trino. Скоро мы ее переведем на русский язык и дополним полезными примерами.
Apache Iceberg is an open table format for huge analytic datasets. The Iceberg connector allows querying data stored in files written in Iceberg format, as defined in the Iceberg Table Spec. The connector supports Apache Iceberg table spec versions 1 and 2.
The table state is maintained in metadata files. All changes to table state create a new metadata file and replace the old metadata with an atomic swap. The table metadata file tracks the table schema, partitioning configuration, custom properties, and snapshots of the table contents.
Iceberg data files are stored in either Parquet, ORC, or Avro format, as
determined by the format
property in the table definition.
Iceberg is designed to improve on the known scalability limitations of Hive, which stores table metadata in a metastore that is backed by a relational database such as MySQL. It tracks partition locations in the metastore, but not individual data files. Trino queries using the Hive коннектор must first call the metastore to get partition locations, then call the underlying file system to list all data files inside each partition, and then read metadata from each data file.
Since Iceberg stores the paths to data files in the metadata files, it only consults the underlying file system for files that must be read.
Requirements#
To use Iceberg, you need:
Network access from the Trino coordinator and workers to the distributed object storage.
Access to a Hive metastore service (HMS), an AWS Glue catalog, a JDBC catalog, a REST catalog, a Nessie server, or a Snowflake catalog.
Data files stored in the file formats Parquet(default), ORC, or Avro on a supported file system.
General configuration#
To configure the Iceberg connector, create a catalog properties file
etc/catalog/example.properties
that references the iceberg
connector.
The Hive metastore catalog is the default implementation.
You must select and configure one of the supported file systems.
connector.name=iceberg
hive.metastore.uri=thrift://example.net:9083
fs.x.enabled=true
Replace the fs.x.enabled
configuration property with the desired file system.
Other metadata catalog types as listed in the requirements section of this topic are available. Each metastore type has specific configuration properties along with general metastore configuration properties.
The following configuration properties are independent of which catalog implementation is used:
Property name |
Description |
Default |
---|---|---|
|
Define the metastore type to use. Possible values are:
|
|
|
Define the data storage file format for Iceberg tables. Possible values are:
|
|
|
The compression codec used when writing files. Possible values are:
|
|
|
Read file sizes from metadata instead of file system. This property must only be used as a workaround for this issue. The problem was fixed in Iceberg version 0.11.0. |
|
|
Maximum number of partitions handled per writer. |
|
|
Target maximum size of written files; the actual size may be larger. |
|
|
Use randomized, unique table locations. |
|
|
Maximum duration to wait for completion of dynamic filters during split generation. |
|
|
Whether schema locations are deleted when Trino can’t determine whether they contain external files. |
|
|
A decimal value in the range |
0.05 |
|
Enable Table statistics. The equivalent catalog session
property is |
|
|
Enable statistics collection with ANALYZE and use of extended
statistics. The equivalent catalog session property is
|
|
|
Enable collection of extended statistics for write operations. The
equivalent catalog session property is
|
|
|
Enable projection pushdown |
|
|
Catalog to redirect to when a Hive table is referenced. |
|
|
Schema for creating materialized views storage tables. When this property is
not configured, storage tables are created in the same schema as the
materialized view definition. When the |
Empty |
|
Enable to allow user to call |
|
|
Set to |
|
|
Specify the list of schemas for which Trino can enforce that queries use a
filter on partition keys for source tables. Equivalent session property is
|
|
|
Set to |
|
|
Set to |
|
Fault-tolerant execution support#
The connector supports Fault-tolerant execution of query processing. Read and write operations are both supported with any retry policy.
File system access configuration#
The connector supports accessing the following file systems:
You must enable and configure the specific file system access. Legacy support is not recommended and will be removed.
Type mapping#
The connector reads and writes data into the supported data file formats Avro, ORC, and Parquet, following the Iceberg specification.
Because Trino and Iceberg each support types that the other does not, this connector modifies some types when reading or writing data. Data types may not map the same way in both directions between Trino and the data source. Refer to the following sections for type mapping in each direction.
The Iceberg specification includes supported data types and the mapping to the formating in the Avro, ORC, or Parquet files:
Iceberg to Trino type mapping#
The connector maps Iceberg types to the corresponding Trino types according to the following table:
Iceberg type |
Trino type |
---|---|
|
|
|
|
|
|
|
|
|
|
|
|
|
|
|
|
|
|
|
|
|
|
|
|
|
|
|
|
|
|
|
|
|
|
No other types are supported.
Trino to Iceberg type mapping#
The connector maps Trino types to the corresponding Iceberg types according to the following table:
Trino type |
Iceberg type |
---|---|
|
|
|
|
|
|
|
|
|
|
|
|
|
|
|
|
|
|
|
|
|
|
|
|
|
|
|
|
|
|
|
|
No other types are supported.
Security#
Kerberos authentication#
The Iceberg connector supports Kerberos authentication for the Hive metastore and HDFS and is configured using the same parameters as the Hive connector. Find more information in the HDFS file system support section.
SQL support#
This connector provides read access and write access to data and metadata in Iceberg. In addition to the globally available and read operation statements, the connector supports the following features:
Basic usage examples#
The connector supports creating schemas. You can create a schema with or without a specified location.
You can create a schema with the CREATE SCHEMA statement and the
location
schema property. The tables in this schema, which have no explicit
location
set in CREATE TABLE statement, are located in a
subdirectory under the directory corresponding to the schema location.
Create a schema on S3:
CREATE SCHEMA example.example_s3_schema
WITH (location = 's3://my-bucket/a/path/');
Create a schema on an S3-compatible object storage such as MinIO:
CREATE SCHEMA example.example_s3a_schema
WITH (location = 's3a://my-bucket/a/path/');
Create a schema on HDFS:
CREATE SCHEMA example.example_hdfs_schema
WITH (location='hdfs://hadoop-master:9000/user/hive/warehouse/a/path/');
Optionally, on HDFS, the location can be omitted:
CREATE SCHEMA example.example_hdfs_schema;
The Iceberg connector supports creating tables using the CREATE TABLE syntax. Optionally, specify the table properties supported by this connector:
CREATE TABLE example_table (
c1 INTEGER,
c2 DATE,
c3 DOUBLE
)
WITH (
format = 'PARQUET',
partitioning = ARRAY['c1', 'c2'],
sorted_by = ARRAY['c3'],
location = 's3://my-bucket/a/path/'
);
When the location
table property is omitted, the content of the table is
stored in a subdirectory under the directory corresponding to the schema
location.
The Iceberg connector supports creating tables using the CREATE TABLE AS with SELECT syntax:
CREATE TABLE tiny_nation
WITH (
format = 'PARQUET'
)
AS
SELECT *
FROM nation
WHERE nationkey < 10;
Another flavor of creating tables with CREATE TABLE AS is with VALUES syntax:
CREATE TABLE yearly_clicks (
year,
clicks
)
WITH (
partitioning = ARRAY['year']
)
AS VALUES
(2021, 10000),
(2022, 20000);
Procedures#
Use the CALL statement to perform data manipulation or
administrative tasks. Procedures are available in the system schema of each
catalog. The following code snippet displays how to call the
example_procedure
in the examplecatalog
catalog:
CALL examplecatalog.system.example_procedure()
Register table#
The connector can register existing Iceberg tables into the metastore if
iceberg.register-table-procedure.enabled
is set to true
for the catalog.
The procedure system.register_table
allows the caller to register an
existing Iceberg table in the metastore, using its existing metadata and data
files:
CALL example.system.register_table(schema_name => 'testdb', table_name => 'customer_orders', table_location => 'hdfs://hadoop-master:9000/user/hive/warehouse/customer_orders-581fad8517934af6be1857a903559d44')
In addition, you can provide a file name to register a table with specific metadata. This may be used to register the table with some specific table state, or may be necessary if the connector cannot automatically figure out the metadata version to use:
CALL example.system.register_table(schema_name => 'testdb', table_name => 'customer_orders', table_location => 'hdfs://hadoop-master:9000/user/hive/warehouse/customer_orders-581fad8517934af6be1857a903559d44', metadata_file_name => '00003-409702ba-4735-4645-8f14-09537cc0b2c8.metadata.json')
To prevent unauthorized users from accessing data, this procedure is disabled by
default. The procedure is enabled only when
iceberg.register-table-procedure.enabled
is set to true
.
Unregister table#
The connector can remove existing Iceberg tables from the metastore. Once unregistered, you can no longer query the table from Trino.
The procedure system.unregister_table
allows the caller to unregister an
existing Iceberg table from the metastores without deleting the data:
CALL example.system.unregister_table(schema_name => 'testdb', table_name => 'customer_orders')
Migrate table#
The connector can read from or write to Hive tables that have been migrated to Iceberg.
Use the procedure system.migrate
to move a table from the Hive format to the
Iceberg format, loaded with the source’s data files. Table schema, partitioning,
properties, and location are copied from the source table. A bucketed Hive table
will be migrated as a non-bucketed Iceberg table. The data files in the Hive table
must use the Parquet, ORC, or Avro file format.
The procedure must be called for a specific catalog example
with the
relevant schema and table names supplied with the required parameters
schema_name
and table_name
:
CALL example.system.migrate(
schema_name => 'testdb',
table_name => 'customer_orders')
Migrate fails if any table partition uses an unsupported file format.
In addition, you can provide a recursive_directory
argument to migrate a
Hive table that contains subdirectories:
CALL example.system.migrate(
schema_name => 'testdb',
table_name => 'customer_orders',
recursive_directory => 'true')
The default value is fail
, which causes the migrate procedure to throw an
exception if subdirectories are found. Set the value to true
to migrate
nested directories, or false
to ignore them.
Data management#
The DML functionality includes support for INSERT
,
UPDATE
, DELETE
, and MERGE
statements.
Deletion by partition#
For partitioned tables, the Iceberg connector supports the deletion of entire
partitions if the WHERE
clause specifies filters only on the
identity-transformed partitioning columns, that can match entire partitions.
Given the table definition from Partitioned Tables
section, the following SQL statement deletes all partitions for which
country
is US
:
DELETE FROM example.testdb.customer_orders
WHERE country = 'US'
A partition delete is performed if the WHERE
clause meets these conditions.
Row level deletion#
Tables using v2 of the Iceberg specification support deletion of individual rows by writing position delete files.
Schema and table management#
The Схемы и таблицы functionality includes support for:
Schema evolution#
Iceberg supports schema evolution, with safe column add, drop, reorder, and rename operations, including in nested structures.
Iceberg supports updating column types only for widening operations:
INTEGER
toBIGINT
REAL
toDOUBLE
DECIMAL(p,s)
toDECIMAL(p2,s)
whenp2
>p
(scale cannot change)
Partitioning can also be changed and the connector can still query data created before the partitioning change.
ALTER TABLE EXECUTE#
The connector supports the following commands for use with ALTER TABLE EXECUTE.
optimize#
The optimize
command is used for rewriting the content of the specified
table so that it is merged into fewer but larger files. If the table is
partitioned, the data compaction acts separately on each partition selected for
optimization. This operation improves read performance.
All files with a size below the optional file_size_threshold
parameter
(default value for the threshold is 100MB
) are merged:
ALTER TABLE test_table EXECUTE optimize
The following statement merges files in a table that are under 128 megabytes in size:
ALTER TABLE test_table EXECUTE optimize(file_size_threshold => '128MB')
You can use a WHERE
clause with the columns used to partition the table
to filter which partitions are optimized:
ALTER TABLE test_partitioned_table EXECUTE optimize
WHERE partition_key = 1
You can use a more complex WHERE
clause to narrow down the scope of the
optimize
procedure. The following example casts the timestamp values to
dates, and uses a comparison to only optimize partitions with data from the year
2022 or newer:
ALTER TABLE test_table EXECUTE optimize
WHERE CAST(timestamp_tz AS DATE) > DATE '2021-12-31'
expire_snapshots#
The expire_snapshots
command removes all snapshots and all related metadata
and data files. Regularly expiring snapshots is recommended to delete data files
that are no longer needed, and to keep the size of table metadata small. The
procedure affects all snapshots that are older than the time period configured
with the retention_threshold
parameter.
expire_snapshots
can be run as follows:
ALTER TABLE test_table EXECUTE expire_snapshots(retention_threshold => '7d')
The value for retention_threshold
must be higher than or equal to
iceberg.expire_snapshots.min-retention
in the catalog, otherwise the
procedure fails with a similar message: Retention specified (1.00d) is shorter than the minimum retention configured in the system (7.00d)
. The default value
for this property is 7d
.
remove_orphan_files#
The remove_orphan_files
command removes all files from a table’s data
directory that are not linked from metadata files and that are older than the
value of retention_threshold
parameter. Deleting orphan files from time to
time is recommended to keep size of a table’s data directory under control.
remove_orphan_files
can be run as follows:
ALTER TABLE test_table EXECUTE remove_orphan_files(retention_threshold => '7d')
The value for retention_threshold
must be higher than or equal to
iceberg.remove_orphan_files.min-retention
in the catalog otherwise the
procedure fails with a similar message: Retention specified (1.00d) is shorter than the minimum retention configured in the system (7.00d)
. The default value
for this property is 7d
.
drop_extended_stats#
The drop_extended_stats
command removes all extended statistics information
from the table.
drop_extended_stats
can be run as follows:
ALTER TABLE test_table EXECUTE drop_extended_stats
ALTER TABLE SET PROPERTIES#
The connector supports modifying the properties on existing tables using ALTER TABLE SET PROPERTIES.
The following table properties can be updated after a table is created:
format
format_version
partitioning
sorted_by
For example, to update a table from v1 of the Iceberg specification to v2:
ALTER TABLE table_name SET PROPERTIES format_version = 2;
Or to set the column my_new_partition_column
as a partition column on a
table:
ALTER TABLE table_name SET PROPERTIES partitioning = ARRAY[<existing partition columns>, 'my_new_partition_column'];
The current values of a table’s properties can be shown using SHOW CREATE TABLE.
Table properties#
Table properties supply or set metadata for the underlying tables. This is key for CREATE TABLE AS statements. Table properties are passed to the connector using a WITH clause.
Property name |
Description |
---|---|
|
Optionally specifies the format of table data files; either |
|
Optionally specifies table partitioning. If a table is partitioned by
columns |
|
The sort order to be applied during writes to the content of
each file written to the table. If the table files are sorted by columns
|
|
Optionally specifies the file system location URI for the table. |
|
Optionally specifies the format version of the Iceberg specification to use
for new tables; either |
|
Comma-separated list of columns to use for ORC bloom filter. It improves the
performance of queries using Equality and IN predicates when reading ORC
files. Requires ORC format. Defaults to |
|
The ORC bloom filters false positive probability. Requires ORC format.
Defaults to |
|
Comma-separated list of columns to use for Parquet bloom filter. It improves
the performance of queries using Equality and IN predicates when reading
Parquet files. Requires Parquet format. Defaults to |
The table definition below specifies to use Parquet files, partitioning by columns
c1
and c2
, and a file system location of
/var/example_tables/test_table
:
CREATE TABLE test_table (
c1 INTEGER,
c2 DATE,
c3 DOUBLE)
WITH (
format = 'PARQUET',
partitioning = ARRAY['c1', 'c2'],
location = '/var/example_tables/test_table')
The table definition below specifies to use ORC files, bloom filter index by columns
c1
and c2
, fpp is 0.05, and a file system location of
/var/example_tables/test_table
:
CREATE TABLE test_table (
c1 INTEGER,
c2 DATE,
c3 DOUBLE)
WITH (
format = 'ORC',
location = '/var/example_tables/test_table',
orc_bloom_filter_columns = ARRAY['c1', 'c2'],
orc_bloom_filter_fpp = 0.05)
The table definition below specifies to use Avro files, partitioning
by child1
field in parent
column:
CREATE TABLE test_table (
data INTEGER,
parent ROW(child1 DOUBLE, child2 INTEGER))
WITH (
format = 'AVRO',
partitioning = ARRAY['"parent.child1"'])
Metadata tables#
The connector exposes several metadata tables for each Iceberg table. These metadata tables contain information about the internal structure of the Iceberg table. You can query each metadata table by appending the metadata table name to the table name:
SELECT * FROM "test_table$properties"
$properties
table#
The $properties
table provides access to general information about Iceberg
table configuration and any additional metadata key/value pairs that the table
is tagged with.
You can retrieve the properties of the current snapshot of the Iceberg table
test_table
by using the following query:
SELECT * FROM "test_table$properties"
key | value |
-----------------------+----------+
write.format.default | PARQUET |
$history
table#
The $history
table provides a log of the metadata changes performed on the
Iceberg table.
You can retrieve the changelog of the Iceberg table test_table
by using the
following query:
SELECT * FROM "test_table$history"
made_current_at | snapshot_id | parent_id | is_current_ancestor
----------------------------------+----------------------+----------------------+--------------------
2022-01-10 08:11:20 Europe/Vienna | 8667764846443717831 | <null> | true
2022-01-10 08:11:34 Europe/Vienna | 7860805980949777961 | 8667764846443717831 | true
The output of the query has the following columns:
Name |
Type |
Description |
---|---|---|
|
|
The time when the snapshot became active. |
|
|
The identifier of the snapshot. |
|
|
The identifier of the parent snapshot. |
|
|
Whether or not this snapshot is an ancestor of the current snapshot. |
$metadata_log_entries
table#
The $metadata_log_entries
table provides a view of metadata log entries
of the Iceberg table.
You can retrieve the information about the metadata log entries of the Iceberg
table test_table
by using the following query:
SELECT * FROM "test_table$metadata_log_entries"
timestamp | file | latest_snapshot_id | latest_schema_id | latest_sequence_number
---------------------------------------+----------------------------------------------------------------------------------------------------------------------------+---------------------+------------------+------------------------
2024-01-16 15:55:31.172 Europe/Vienna | hdfs://hadoop-master:9000/user/hive/warehouse/test_table/metadata/00000-39174715-be2a-48fa-9949-35413b8b736e.metadata.json | 1221802298419195590 | 0 | 1
2024-01-16 17:19:56.118 Europe/Vienna | hdfs://hadoop-master:9000/user/hive/warehouse/test_table/metadata/00001-e40178c9-271f-4a96-ad29-eed5e7aef9b0.metadata.json | 7124386610209126943 | 0 | 2
The output of the query has the following columns:
Name |
Type |
Description |
---|---|---|
|
|
The time when the metadata was created. |
|
|
The location of the metadata file. |
|
|
The identifier of the latest snapshot when the metadata was updated. |
|
|
The identifier of the latest schema when the metadata was updated. |
|
|
The data sequence number of the metadata file. |
$snapshots
table#
The $snapshots
table provides a detailed view of snapshots of the Iceberg
table. A snapshot consists of one or more file manifests, and the complete table
contents are represented by the union of all the data files in those manifests.
You can retrieve the information about the snapshots of the Iceberg table
test_table
by using the following query:
SELECT * FROM "test_table$snapshots"
committed_at | snapshot_id | parent_id | operation | manifest_list | summary
----------------------------------+----------------------+----------------------+--------------------+------------------------------------------------------------------------------------------------------------------------------------------+----------------------------------------------------------------------------------------------------------------------------------------------------------------------------------------------------------------------------
2022-01-10 08:11:20 Europe/Vienna | 8667764846443717831 | <null> | append | hdfs://hadoop-master:9000/user/hive/warehouse/test_table/metadata/snap-8667764846443717831-1-100cf97e-6d56-446e-8961-afdaded63bc4.avro | {changed-partition-count=0, total-equality-deletes=0, total-position-deletes=0, total-delete-files=0, total-files-size=0, total-records=0, total-data-files=0}
2022-01-10 08:11:34 Europe/Vienna | 7860805980949777961 | 8667764846443717831 | append | hdfs://hadoop-master:9000/user/hive/warehouse/test_table/metadata/snap-7860805980949777961-1-faa19903-1455-4bb8-855a-61a1bbafbaa7.avro | {changed-partition-count=1, added-data-files=1, total-equality-deletes=0, added-records=1, total-position-deletes=0, added-files-size=442, total-delete-files=0, total-files-size=442, total-records=1, total-data-files=1}
The output of the query has the following columns:
Name |
Type |
Description |
---|---|---|
|
|
The time when the snapshot became active. |
|
|
The identifier for the snapshot. |
|
|
The identifier for the parent snapshot. |
|
|
The type of operation performed on the Iceberg table. The supported operation types in Iceberg are:
|
|
|
The list of Avro manifest files containing the detailed information about the snapshot changes. |
|
|
A summary of the changes made from the previous snapshot to the current snapshot. |
$manifests
table#
The $manifests
table provides a detailed overview of the manifests
corresponding to the snapshots performed in the log of the Iceberg table.
You can retrieve the information about the manifests of the Iceberg table
test_table
by using the following query:
SELECT * FROM "test_table$manifests"
path | length | partition_spec_id | added_snapshot_id | added_data_files_count | added_rows_count | existing_data_files_count | existing_rows_count | deleted_data_files_count | deleted_rows_count | partitions
----------------------------------------------------------------------------------------------------------------+-----------------+----------------------+-----------------------+-------------------------+------------------+-----------------------------+---------------------+-----------------------------+--------------------+----------------------------------------------------------------------------------------------------------------------------------------------------------------------
hdfs://hadoop-master:9000/user/hive/warehouse/test_table/metadata/faa19903-1455-4bb8-855a-61a1bbafbaa7-m0.avro | 6277 | 0 | 7860805980949777961 | 1 | 100 | 0 | 0 | 0 | 0 | {{contains_null=false, contains_nan= false, lower_bound=1, upper_bound=1},{contains_null=false, contains_nan= false, lower_bound=2021-01-12, upper_bound=2021-01-12}}
The output of the query has the following columns:
Name |
Type |
Description |
---|---|---|
|
|
The manifest file location. |
|
|
The manifest file length. |
|
|
The identifier for the partition specification used to write the manifest file. |
|
|
The identifier of the snapshot during which this manifest entry has been added. |
|
|
The number of data files with status |
|
|
The total number of rows in all data files with status |
|
|
The number of data files with status |
|
|
The total number of rows in all data files with status |
|
|
The number of data files with status |
|
|
The total number of rows in all data files with status |
|
|
Partition range metadata. |
$partitions
table#
The $partitions
table provides a detailed overview of the partitions of the
Iceberg table.
You can retrieve the information about the partitions of the Iceberg table
test_table
by using the following query:
SELECT * FROM "test_table$partitions"
partition | record_count | file_count | total_size | data
-----------------------+---------------+---------------+---------------+------------------------------------------------------
{c1=1, c2=2021-01-12} | 2 | 2 | 884 | {c3={min=1.0, max=2.0, null_count=0, nan_count=NULL}}
{c1=1, c2=2021-01-13} | 1 | 1 | 442 | {c3={min=1.0, max=1.0, null_count=0, nan_count=NULL}}
The output of the query has the following columns:
Name |
Type |
Description |
---|---|---|
|
|
A row that contains the mapping of the partition column names to the partition column values. |
|
|
The number of records in the partition. |
|
|
The number of files mapped in the partition. |
|
|
The size of all the files in the partition. |
|
|
Partition range metadata. |
$files
table#
The $files
table provides a detailed overview of the data files in current
snapshot of the Iceberg table.
To retrieve the information about the data files of the Iceberg table
test_table
, use the following query:
SELECT * FROM "test_table$files"
content | file_path | record_count | file_format | file_size_in_bytes | column_sizes | value_counts | null_value_counts | nan_value_counts | lower_bounds | upper_bounds | key_metadata | split_offsets | equality_ids
----------+-------------------------------------------------------------------------------------------------------------------------------+-----------------+---------------+----------------------+----------------------+-------------------+--------------------+-------------------+-----------------------------+-----------------------------+----------------+----------------+---------------
0 | hdfs://hadoop-master:9000/user/hive/warehouse/test_table/data/c1=3/c2=2021-01-14/af9872b2-40f3-428f-9c87-186d2750d84e.parquet | 1 | PARQUET | 442 | {1=40, 2=40, 3=44} | {1=1, 2=1, 3=1} | {1=0, 2=0, 3=0} | <null> | {1=3, 2=2021-01-14, 3=1.3} | {1=3, 2=2021-01-14, 3=1.3} | <null> | <null> | <null>
The output of the query has the following columns:
Name |
Type |
Description |
---|---|---|
|
|
Type of content stored in the file. The supported content types in Iceberg are:
|
|
|
The data file location. |
|
|
The format of the data file. |
|
|
The number of entries contained in the data file. |
|
|
The data file size |
|
|
Mapping between the Iceberg column ID and its corresponding size in the file. |
|
|
Mapping between the Iceberg column ID and its corresponding count of entries in the file. |
|
|
Mapping between the Iceberg column ID and its corresponding count of |
|
|
Mapping between the Iceberg column ID and its corresponding count of non- numerical values in the file. |
|
|
Mapping between the Iceberg column ID and its corresponding lower bound in the file. |
|
|
Mapping between the Iceberg column ID and its corresponding upper bound in the file. |
|
|
Metadata about the encryption key used to encrypt this file, if applicable. |
|
|
List of recommended split locations. |
|
|
The set of field IDs used for equality comparison in equality delete files. |
$refs
table#
The $refs
table provides information about Iceberg references including
branches and tags.
You can retrieve the references of the Iceberg table test_table
by using the
following query:
SELECT * FROM "test_table$refs"
name | type | snapshot_id | max_reference_age_in_ms | min_snapshots_to_keep | max_snapshot_age_in_ms |
----------------+--------+-------------+-------------------------+-----------------------+------------------------+
example_tag | TAG | 10000000000 | 10000 | null | null |
example_branch | BRANCH | 20000000000 | 20000 | 2 | 30000 |
The output of the query has the following columns:
Name |
Type |
Description |
---|---|---|
|
|
Name of the reference. |
|
|
Type of the reference, either |
|
|
The snapshot ID of the reference. |
|
|
The maximum age of the reference before it could be expired. |
|
|
For branch only, the minimum number of snapshots to keep in a branch. |
|
|
For branch only, the max snapshot age allowed in a branch. Older snapshots in the branch will be expired. |
Metadata columns#
In addition to the defined columns, the Iceberg connector automatically exposes path metadata as a hidden column in each table:
$path
: Full file system path name of the file for this row$file_modified_time
: Timestamp of the last modification of the file for this row
You can use these columns in your SQL statements like any other column. This can be selected directly, or used in conditional statements. For example, you can inspect the file path for each record:
SELECT *, "$path", "$file_modified_time"
FROM example.web.page_views;
Retrieve all records that belong to a specific file using "$path"
filter:
SELECT *
FROM example.web.page_views
WHERE "$path" = '/usr/iceberg/table/web.page_views/data/file_01.parquet'
Retrieve all records that belong to a specific file using
"$file_modified_time"
filter:
SELECT *
FROM example.web.page_views
WHERE "$file_modified_time" = CAST('2022-07-01 01:02:03.456 UTC' AS TIMESTAMP WITH TIME ZONE)
DROP TABLE#
The Iceberg connector supports dropping a table by using the DROP TABLE syntax. When the command succeeds, both the data of the Iceberg table and also the information related to the table in the metastore service are removed. Dropping tables that have their data/metadata stored in a different location than the table’s corresponding base directory on the object store is not supported.
COMMENT#
The Iceberg connector supports setting comments on the following objects:
tables
views
table columns
materialized view columns
The COMMENT
option is supported on both the table and the table columns for
the CREATE TABLE operation.
The COMMENT
option is supported for adding table columns through the
ALTER TABLE operations.
The connector supports the command COMMENT for setting comments on existing entities.
Partitioned tables#
Iceberg supports partitioning by specifying transforms over the table columns. A partition is created for each unique tuple value produced by the transforms. Identity transforms are simply the column name. Other transforms are:
Transform |
Description |
---|---|
|
A partition is created for each year. The partition value is the integer
difference in years between |
|
A partition is created for each month of each year. The partition value is
the integer difference in months between |
|
A partition is created for each day of each year. The partition value is the
integer difference in days between |
|
A partition is created hour of each day. The partition value is a timestamp with the minutes and seconds set to zero. |
|
The data is hashed into the specified number of buckets. The partition value
is an integer hash of |
|
The partition value is the first |
In this example, the table is partitioned by the month of order_date
, a hash
of account_number
(with 10 buckets), and country
:
CREATE TABLE example.testdb.customer_orders (
order_id BIGINT,
order_date DATE,
account_number BIGINT,
customer VARCHAR,
country VARCHAR)
WITH (partitioning = ARRAY['month(order_date)', 'bucket(account_number, 10)', 'country'])
Sorted tables#
The connector supports sorted files as a performance improvement. Data is sorted during writes within each file based on the specified array of one or more columns.
Sorting is particularly beneficial when the sorted columns show a high cardinality and are used as a filter for selective reads.
The sort order is configured with the sorted_by
table property. Specify an
array of one or more columns to use for sorting when creating the table. The
following example configures the order_date
column of the orders
table
in the customers
schema in the example
catalog:
CREATE TABLE example.customers.orders (
order_id BIGINT,
order_date DATE,
account_number BIGINT,
customer VARCHAR,
country VARCHAR)
WITH (sorted_by = ARRAY['order_date'])
Sorting can be combined with partitioning on the same column. For example:
CREATE TABLE example.customers.orders (
order_id BIGINT,
order_date DATE,
account_number BIGINT,
customer VARCHAR,
country VARCHAR)
WITH (
partitioning = ARRAY['month(order_date)'],
sorted_by = ARRAY['order_date']
)
You can disable sorted writing with the session property
sorted_writing_enabled
set to false
.
Using snapshots#
Iceberg supports a snapshot model of data, where table snapshots are identified by a snapshot ID.
The connector provides a system table exposing snapshot information for every
Iceberg table. Snapshots are identified by BIGINT
snapshot IDs. For example,
you can find the snapshot IDs for the customer_orders
table by running the
following query:
SELECT snapshot_id
FROM example.testdb."customer_orders$snapshots"
ORDER BY committed_at DESC
Replace tables#
The connector supports replacing an existing table, as an atomic operation. Atomic table replacement creates a new snapshot with the new table definition as part of the table history.
To replace a table, use CREATE OR REPLACE TABLE
or
CREATE OR REPLACE TABLE AS
.
Earlier snapshots of the table can be queried through Time travel queries.
In the following example, a table example_table
can be replaced by a
completely new definition and data from the source table:
CREATE OR REPLACE TABLE example_table
WITH (sorted_by = ARRAY['a'])
AS SELECT * FROM another_table;
Time travel queries#
The connector offers the ability to query historical data. This allows you to query the table as it was when a previous snapshot of the table was taken, even if the data has since been modified or deleted.
The historical data of the table can be retrieved by specifying the snapshot identifier corresponding to the version of the table to be retrieved:
SELECT *
FROM example.testdb.customer_orders FOR VERSION AS OF 8954597067493422955
A different approach of retrieving historical data is to specify a point in time in the past, such as a day or week ago. The latest snapshot of the table taken before or at the specified timestamp in the query is internally used for providing the previous state of the table:
SELECT *
FROM example.testdb.customer_orders FOR TIMESTAMP AS OF TIMESTAMP '2022-03-23 09:59:29.803 Europe/Vienna'
The connector allows to create a new snapshot through Iceberg’s replace table.
CREATE OR REPLACE TABLE example.testdb.customer_orders AS
SELECT *
FROM example.testdb.customer_orders FOR TIMESTAMP AS OF TIMESTAMP '2022-03-23 09:59:29.803 Europe/Vienna'
You can use a date to specify a point a time in the past for using a snapshot of a table in a query.
Assuming that the session time zone is Europe/Vienna
the following queries are equivalent:
SELECT *
FROM example.testdb.customer_orders FOR TIMESTAMP AS OF DATE '2022-03-23'
SELECT *
FROM example.testdb.customer_orders FOR TIMESTAMP AS OF TIMESTAMP '2022-03-23 00:00:00'
SELECT *
FROM example.testdb.customer_orders FOR TIMESTAMP AS OF TIMESTAMP '2022-03-23 00:00:00.000 Europe/Vienna'
Iceberg supports named references of snapshots via branches and tags. Time travel can be performed to branches and tags in the table.
SELECT *
FROM example.testdb.customer_orders FOR VERSION AS OF 'historical-tag'
SELECT *
FROM example.testdb.customer_orders FOR VERSION AS OF 'test-branch'
Rolling back to a previous snapshot#
Use the $snapshots
metadata table to determine the latest snapshot ID of the
table like in the following query:
SELECT snapshot_id
FROM example.testdb."customer_orders$snapshots"
ORDER BY committed_at DESC LIMIT 1
The procedure system.rollback_to_snapshot
allows the caller to roll back the
state of the table to a previous snapshot id:
CALL example.system.rollback_to_snapshot('testdb', 'customer_orders', 8954597067493422955)
NOT NULL
column constraint#
The Iceberg connector supports setting NOT NULL
constraints on the table
columns.
The NOT NULL
constraint can be set on the columns, while creating tables by
using the CREATE TABLE syntax:
CREATE TABLE example_table (
year INTEGER NOT NULL,
name VARCHAR NOT NULL,
age INTEGER,
address VARCHAR
);
When trying to insert/update data in the table, the query fails if trying to set
NULL
value on a column having the NOT NULL
constraint.
Materialized views#
The Iceberg connector supports Материализованные представления (materialized view). In the underlying system, each materialized view consists of a view definition and an Iceberg storage table. The storage table name is stored as a materialized view property. The data is stored in that storage table.
You can use the Table properties to control the created storage
table and therefore the layout and performance. For example, you can use the
following clause with CREATE MATERIALIZED VIEW to use the ORC format
for the data files and partition the storage per day using the column
event_date
:
WITH ( format = 'ORC', partitioning = ARRAY['event_date'] )
By default, the storage table is created in the same schema as the materialized
view definition. The iceberg.materialized-views.storage-schema
catalog
configuration property or storage_schema
materialized view property can be
used to specify the schema where the storage table is created.
Creating a materialized view does not automatically populate it with data. You must run REFRESH MATERIALIZED VIEW to populate data in the materialized view.
Updating the data in the materialized view can be achieved using the REFRESH MATERIALIZED VIEW
command. This operation may perform either an incremental or
a full refresh, depending on the complexity of the materialized view definition
and the snapshot history of the source tables. For a full refresh, the operation
deletes the data from the storage table, and inserts the data that is the result
of executing the materialized view query into the existing table. For
incremental refresh, the existing data is not deleted from the storage table and
only the delta records are processed from the source tables and appended into
the storage table as needed. In both cases, data is replaced or appended
atomically, so users can continue to query the materialized view while it is
being refreshed. Refreshing a materialized view also stores the snapshot-ids of
all Iceberg tables that are part of the materialized view’s query in the
materialized view metadata. When the materialized view is queried, the
snapshot-ids are used to check if the data in the storage table is up to date.
Materialized views that use non-Iceberg tables in the query show the default behavior around grace periods. If all tables are Iceberg tables, the connector can determine if the data has not changed and continue to use the data from the storage tables, even after the grace period expired.
Dropping a materialized view with DROP MATERIALIZED VIEW removes the definition and the storage table.
Table functions#
The connector supports the table functions described in the following sections.
table_changes#
Allows reading row-level changes between two versions of an Iceberg table.
The following query shows an example of displaying the changes of the t1
table in the default
schema in the current catalog.
All changes between the start and end snapshots are returned.
SELECT
*
FROM
TABLE(
system.table_changes(
schema_name => 'default',
table_name => 't1',
start_snapshot_id => 6541165659943306573,
end_snapshot_id => 6745790645714043599
)
);
The function takes the following required parameters:
schema_name
Name of the schema for which the function is called.
table_name
Name of the table for which the function is called.
start_snapshot_id
The identifier of the exclusive starting snapshot.
end_snapshot_id
The identifier of the inclusive end snapshot.
Use the $snapshots
metadata table to determine the snapshot IDs of the
table.
The function returns the columns present in the table, and the following values for each change:
_change_type
The type of change that occurred. Possible values are
insert
anddelete
.
_change_version_id
The identifier of the snapshot in which the change occurred.
_change_timestamp
Timestamp when the snapshot became active.
_change_ordinal
Order number of the change, useful for sorting the results.
Example:
Create a table:
CREATE TABLE test_schema.pages (page_url VARCHAR, domain VARCHAR, views INTEGER);
Insert some data:
INSERT INTO test_schema.pages
VALUES
('url1', 'domain1', 1),
('url2', 'domain2', 2),
('url3', 'domain1', 3);
INSERT INTO test_schema.pages
VALUES
('url4', 'domain1', 400),
('url5', 'domain2', 500),
('url6', 'domain3', 2);
Retrieve the snapshot identifiers of the changes performed on the table:
SELECT
snapshot_id,
parent_id,
operation
FROM test_schema."pages$snapshots";
snapshot_id | parent_id | operation
---------------------+---------------------+-----------
2009020668682716382 | NULL | append
2135434251890923160 | 2009020668682716382 | append
3108755571950643966 | 2135434251890923160 | append
(3 rows)
Select the changes performed in the previously-mentioned INSERT
statements:
SELECT
*
FROM
TABLE(
system.table_changes(
schema_name => 'test_schema',
table_name => 'pages',
start_snapshot_id => 2009020668682716382,
end_snapshot_id => 3108755571950643966
)
)
ORDER BY _change_ordinal ASC;
page_url | domain | views | _change_type | _change_version_id | _change_timestamp | _change_ordinal
----------+---------+-------+--------------+---------------------+-----------------------------+-----------------
url1 | domain1 | 1 | insert | 2135434251890923160 | 2024-04-04 21:24:26.105 UTC | 0
url2 | domain2 | 2 | insert | 2135434251890923160 | 2024-04-04 21:24:26.105 UTC | 0
url3 | domain1 | 3 | insert | 2135434251890923160 | 2024-04-04 21:24:26.105 UTC | 0
url4 | domain1 | 400 | insert | 3108755571950643966 | 2024-04-04 21:24:28.318 UTC | 1
url5 | domain2 | 500 | insert | 3108755571950643966 | 2024-04-04 21:24:28.318 UTC | 1
url6 | domain3 | 2 | insert | 3108755571950643966 | 2024-04-04 21:24:28.318 UTC | 1
(6 rows)
Производительность#
Данная секция описывает важные улучшения производительности, реализованные в Iceberg коннекторе.
Локальный дисковый кэш данных#
Коннектор позволяет сохранять часть данных из озера данных на дисках worker-узлов CedrusData для ускорения доступа к ним. Во многих случаях использование локального дискового кэша приводит к кратному ускорению запросов.
При каждом доступе к колонке CedrusData проверяет, были ли закэшированные данные изменены в удаленном источнике. Если обнаружено изменение, локальные данные будут удалены и закэшированы повторно.
CedrusData кэширует метаданные файлов, а также диапазоны записей по мере необходимости. Гранулярность кэширования диапазонов записей зависит от формата:
Для формата Parquet единицей кэширования являются данные колонки внутри row group
Для формата ORC единицей кэширования являются данные колонки внутри stripe
Для включения локального дискового кэша необходимо:
Установить параметр конфигурации каталога
cedrusdata.hive.data-cache.enabled=true
Указать путь к файлу, в котором описаны правила кэширования в JSON формате
Для узлов, которые будут выполнять запросы (все worker-узлы, а также coordinator-узел, запущенный с параметром
node-scheduler.include-coordinator=true
) необходимо также указать путь к директории, в которой CedrusData будет хранить закэшированные данные. Параметр конфигурации:cedrusdata.hive.data-cache.path
Пример конфигурации для координатора, который не выполняет запросы:
cedrusdata.hive.data-cache.enabled=true
cedrusdata.hive.data-cache.rules.file=/path/to/rules.json
Пример конфигурации для worker-узла или координатора, который выполняет запросы (node-scheduler.include-coordinator=true
):
cedrusdata.hive.data-cache.enabled=true
cedrusdata.hive.data-cache.rules.file=/path/to/rules.json
cedrusdata.hive.data-cache.path=file:///path/to/cache/dir
Правила кэширования необходимо задать в отдельном файле в формате:
{
"rules": [
<rule1>,
<rule2>,
...
]
}
Где <rule>
представляет собой отдельное правило в формате:
{
"schema": "<regexp схемы>",
"table": "<regexp таблицы>",
"partition_filter": "<предикат ключа партиционирования таблицы>",
"distribution_mode": "<способ распределения сплитов по узлам>",
"affinity_mode": "<способ привязки сплитов к узлам>",
"affinity_node_count": "<количество узлов на которых может быть обработан сплит>",
"disable_cache": <флаг отключения кэширования>
}
Подробное описание полей правила:
Название |
Описание |
---|---|
|
Обязательное поле.
Задает паттерн для схем в формате Java Pattern.
Например, |
|
Опциональное поле.
Задает паттерн для таблиц в формате Java Pattern.
Например, |
|
Опциональное поле.
Задает фильтр партиции в виде SQL выражения. Выражение может ссылаться на колонки партиции таблицы и использовать стандартные функции.
Выражение не может ссылаться на колонки, которые не входят в ключ партиции, а также содержать подзапросы и вызовы табличных функций.
Вызов функций происходит от имени специального системного пользователя, на которого не распространяются проверки доступа к функциям.
Если значение |
|
Опциональное поле.
Поддерживается только для Hive коннектора.
Задает способ сопоставления сплитов с worker-узлами.
Допустимые значения: |
|
Опциональное поле.
Задает механизм привязки сплита к узлу.
Допустимые значения: |
|
Опциональное поле.
Задает количество узлов на которых может быть выполнен сплит.
Увеличение данного значения приводит к дублированию закэшированных в кластере, но обеспечивает более равномерное распределение нагрузки.
Если |
|
Опциональное поле.
Позволяет отключить кэширование заданных объектов, соответствующих заданным паттернам |
Обработка правил происходит в порядке их указания в файле сверху вниз. Проверка, кэшировать ли данные из текущей таблицы или партиции, завершается, как только найдено первое подходящее правило.
Ниже приведен полный пример файла правил, который включает кэширование для всех таблиц схем s1
и s2
, кроме таблицы s2.excluded_table
,
а также для таблицы s3.partitioned_table
, в которой закэшированы будут только партиции продаж за последний месяц:
{
"rules": [
{
"schema": "s2",
"table": "excluded_table",
"disable_cache": true
},
{
"schema": "s1|s2"
},
{
"schema": "s3",
"table": "partitioned_table",
"partition_filter": "sales_date + interval '1' month <= current_date"
}
]
}
Вы можете изменять содержимое файла без перезапуска узла. Повторное чтение содержимого файла происходит периодически в соответствии с параметром конфигурации
cedrusdata.hive.data-cache.rules.refresh-period
.
Название |
Описание |
Значение по умолчанию |
---|---|---|
|
Использовать ли локальный кэш данных. Параметр сессии: |
|
|
Путь к файлу с правилами кэширования в формате JSON. |
|
|
Как частно повторно считывать правила кэширования из файла, путь к которому задан в |
|
|
Имя часового пояса, в контексте которого происходит вычисление предикатов партиций. |
Имя текущего часового пояса JVM |
|
Размер кэша, в котором хранится решение о кэшировании и скомпилированный предикат партиции (при наличии) для таблиц. Значение 0 отключает кэширование (не рекомендовано). |
1000 |
|
Путь к локальной директории узла, в котором будут сохранены закэшированные данные.
Путь должен быть задан в формате |
|
|
Максимальный размер кэша данных. При превышении размера CedrusData начнет удаление наиболее редко используемых данных. Данный размер учитывает реальный размер данных на диске с учетом возможной компрессии (см. ниже). |
|
|
Максимальное время хранения записи в кэше. По истечении данного времени запись будет удалена из кэша. |
|
|
Режим компрессии закэшированных данных. При отсутствии компрессии данные занимают больше места на диске, но при
этом требуют меньше ресурсов CPU для чтения. При включенной компрессии данные занимают меньше месте на диске, но
каждая операция чтения потребляет больше CPU. Принимайте решение о включении компрессии на основе того, какой
ресурс узла является более дефицитным. Доступные значения: |
|
|
Как часто производить очистку кэша от устаревших записей. Запись считается устаревшей, если истек ее TTL, заданный параметром |
|
|
Сколько записей возвращать движку CedrusData при чтении данных из кэша. Используется для тонкой настройки производительности. В большинстве случаев его изменение не требуется. |
|
|
Максимальное количество операций кэширования удаленных данных. Когда CedrusData обнаруживает, что удаленные данные соответствуют заданным правилам кэширования, но отсутствуют в кэше, происходит асинхронное кэширование данных, которое требует повторное удаленное чтение. Таким образом, при прогреве кэша могут возникать всплески сетевой и дисковой I/O активности, которые могут негативно сказаться на производительности текущих запросов. Для уменьшения негативного эффекта вы можете задать максимальное количество запросов на запись данных в кэш. |
|
|
Размер буфера при чтении данных с диска. Увеличения размера буфера приводит к уменьшению количества IOPS,
требуемых для чтения данных, но увеличивает потребление памяти. Значение по умолчанию должно хорошо
справляться с большинством типичных нагрузок. Настройка данного параметра может быть полезна в облачных
окружениях, которые зачастую ограничивают количество IOPS в секунду. Обратите внимание, что размерность
«килобайт» необходимо указывать как |
|
|
Размер буфера для записи данных на диск. Увеличения размера буфера приводит к уменьшению количества IOPS,
требуемых для записи данных, но увеличивает потребление памяти. Значение по умолчанию должно хорошо
справляться с большинством типичных нагрузок. Настройка данного параметра может быть полезна в облачных
окружениях, которые зачастую ограничивают количество IOPS в секунду. Обратите внимание, что размерность
«килобайт» необходимо указывать как |
|
Для отчистки кэша конкретного каталога воспользуйтесь встроенной процедурой system.cedrusdata.clear_data_cache
.
Единственным аргументом процедуры является название каталога. Следующий запрос очищает кэш каталога my_data_lake
:
CALL system.cedrusdata.clear_data_cache('my_data_lake');
Статистики работы кэша доступны через таблицу JMX коннектор trino.plugin.hive.datacache:name=<имя каталога>,type=hivedatacacheservice
.
Следующий запрос отображает текущие статистики кэша каталога my_data_lake
:
SELECT * FROM jmx."current"."trino.plugin.hive.datacache:name=my_data_lake,type=hivedatacacheservice"
Оптимизация запросов к partitioned таблицам#
Если таблица Iceberg была создана с параметром partitioning
, то CedrusData может использовать информацию о схеме партиционирования для выбора более оптимального плана запроса.
Для оптимизации могут быть использованы трансформации (transform), которые производят значения следующих типов:
BOOLEAN
, INTEGER
, BIGINT
, REAL
, DOUBLE
, DECIMAL
, DATE
, TIME
, TIMESTAMP
, TIMESTAMP WITH TIME ZONE
, VARCHAR
, VARBINARY
, UUID
.
Наибольшее ускорение ожидается для запросов, в которых присутствуют операторы Join
и Aggregation
. Например:
SELECT ...
FROM t1 JOIN t2
ON t1.partitioning_column = t2.other_column
SELECT a, partitioning_column, b, sum(c)
FROM t
GROUP BY a, partitioning_column, b
Ускорение запросов происходит за счет того, что оптимизатор использует информацию о ключе партиционирования для выбора
более быстрого способа выполнения того или иного оператора. Например, для осуществления группировки по двум атрибутам
GROUP BY a, b
CedrusData обычно осуществляет предварительную группировку локально на узлах, после чего пересылает
полученные данные между узлами с помощью оператора Exchange, чтобы осуществить финальную группировку:
Parent
Aggregation[FINAL, groupBy=[a,b]]
Exchange
Aggregation[PARTIAL, groupBy=[a,b]]
TableScan
Если же одна из колонок a
или b
является ключом партиционирования таблицы, оптимизатор CedrusData использует
эту информацию, чтобы осуществить полную группировку локально, тем самым упрощая план запроса:
Parent
Aggregation[FINAL, groupBy=[a,b]]
TableScan
Название |
Описание |
Значение по умолчанию |
---|---|---|
|
Использовать ли информацию о схеме партиционирования таблиц Iceberg для оптимизации запросов.
Параметр сессии: |
|
Автоматическое переписывание запросов на материализованные представления#
Примечание
Данный функционал является экспериментальным и находится в активной разработке.
CedrusData позволяет автоматически переписывать запросы на материализованные представления.
Для включения автоматической перезаписи запросов на материализованные представления
задайте в файле etc/config.properties
параметр конфигурации cedrusdata.optimizer.materialized-view-rewrite.enabled=true
,
или задайте свойство сессии cedrusdata_materialized_views_rewrite_enabled
:
SET SESSION cedrusdata_materialized_views_rewrite_enabled = true;
Если последнее обновление материализованного представления было произведено с помощью Trino или CedrusData до версии 442-3
,
необходимо вызвать команду REFRESH MATERIALIZED VIEW. В противном случае CedrusData не сможет использовать
материализованное представление для автоматической перезаписи запросов.
Например, вы можете создать материализованное представление, которое агрегирует продажи по дате:
CREATE MATERIALIZED VIEW sales_agg AS
SELECT sold_date, sum(amount)
FROM sales
GROUP BY sold_date;
REFRESH MATERIALIZED VIEW sales_agg;
Если пользователь напишет запрос со схожей агрегацией, но дополнительным фильтром:
CREATE MATERIALIZED VIEW sales_agg AS
SELECT sold_date, sum(amount)
FROM sales
GROUP BY sales_date
WHERE sold_date > DATE '2024-01-01'
То запрос будет автоматически переписан на:
SELECT sold_date, sum(amount)
FROM sales_agg
WHERE sold_date > DATE '2024-01-01'
Примечание
В настоящее время CedrusData не может использовать для автоматического переписывания запросов материализованные представления, которые содержат операцию JOIN
.
Данное ограничение будет устранено в следующих версиях продукта.
Table statistics#
The Iceberg connector can collect column statistics using ANALYZE
statement. This can be disabled using iceberg.extended-statistics.enabled
catalog configuration property, or the corresponding
extended_statistics_enabled
session property.
Updating table statistics#
If your queries are complex and include joining large data sets, running ANALYZE on tables may improve query performance by collecting statistical information about the data:
ANALYZE table_name
This query collects statistics for all columns.
On wide tables, collecting statistics for all columns can be expensive. It is
also typically unnecessary - statistics are only useful on specific columns,
like join keys, predicates, or grouping keys. You can specify a subset of
columns to analyzed with the optional columns
property:
ANALYZE table_name WITH (columns = ARRAY['col_1', 'col_2'])
This query collects statistics for columns col_1
and col_2
.
Note that if statistics were previously collected for all columns, they must be dropped using the drop_extended_stats command before re-analyzing.
Table redirection#
Trino offers the possibility to transparently redirect operations on an existing table to the appropriate catalog based on the format of the table and catalog configuration.
In the context of connectors which depend on a metastore service (for example, Hive коннектор, Iceberg коннектор and Delta Lake коннектор), the metastore (Hive metastore service, AWS Glue Data Catalog) can be used to accustom tables with different table formats. Therefore, a metastore database can hold a variety of tables with different table formats.
As a concrete example, let’s use the following simple scenario which makes use of table redirection:
USE example.example_schema;
EXPLAIN SELECT * FROM example_table;
Query Plan
-------------------------------------------------------------------------
Fragment 0 [SOURCE]
...
Output[columnNames = [...]]
│ ...
└─ TableScan[table = another_catalog:example_schema:example_table]
...
The output of the EXPLAIN
statement points out the actual
catalog which is handling the SELECT
query over the table example_table
.
The table redirection functionality works also when using fully qualified names for the tables:
EXPLAIN SELECT * FROM example.example_schema.example_table;
Query Plan
-------------------------------------------------------------------------
Fragment 0 [SOURCE]
...
Output[columnNames = [...]]
│ ...
└─ TableScan[table = another_catalog:example_schema:example_table]
...
Trino offers table redirection support for the following operations:
Table read operations
Table write operations
Table management operations
Trino does not offer view redirection support.
The connector supports redirection from Iceberg tables to Hive tables with the
iceberg.hive-catalog-name
catalog configuration property.
File system cache#
The connector supports configuring and using file system caching.